Harnessing AI to Drive Faster and Smarter Data and Analytics Value Delivery
- Mike Booth
- Mar 21
- 5 min read
The Transformation Imperative
In boardrooms across Australia's financial sector, a quiet revolution is underway. While executives debate the merits of various strategic initiatives, forward-thinking institutions have already begun rewriting the rules of competitive advantage through a powerful combination: advanced data analytics and artificial intelligence.
This isn't merely another technology trend to monitor from afar. The Australian financial landscape is rapidly dividing into two distinct camps: those who are strategically deploying AI to transform their data operations, and those who soon will wish they had.
Consider the evidence: 83% of Australian banking executives are already integrating AI technology into their operations (YouGov). Early adopters aren't just gaining marginal improvements—they're reporting 50% reductions in data migration timeframes, 25% cuts in engineering costs, and dramatically improved visibility into operational metadata.
But realising the benefits is more than just buying the latest technology, it requires adopting new data engineering practices and processes.
Why This Matters to You Now
As a leader the financial services sector, you're likely inundated with technology investment proposals promising transformative returns. The scepticism is understandable. Yet the AI-powered data revolution differs fundamentally from previous cycles of technology hype.
Your competitors aren't merely experimenting with AI—they're systematically deploying it across their data ecosystem to create sustainable advantages that will prove increasingly difficult to overcome:
ANZ Group has established a centralised data and analytics unit alongside an AI 'immersion' centre that will educate 3,000 bank leaders within 12 months
Commonwealth Bank of Australia has halved its data migration timeframe from 18 months to just 9 months through AI-powered automation
A mid-sized Australian bank has reduced "idea-to-deployment" costs by 25% and delivery time by 60% while increasing test coverage to between 50-90%
These aren't speculative future benefits. They're measurable competitive advantages being realised today.
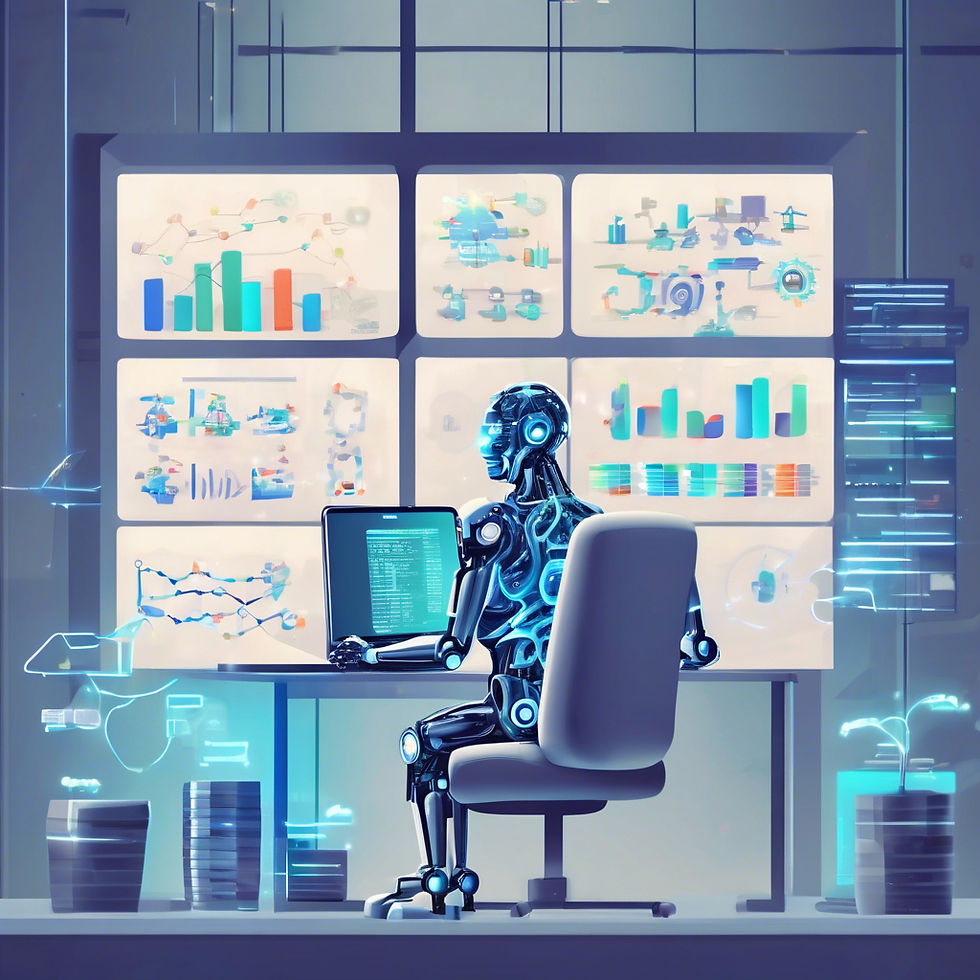
The Hidden Cost of Inaction
The true risk isn't in making the wrong technology investment—it's in maintaining the status quo while the competitive landscape fundamentally shifts.
Your organisation likely possesses vast data assets, yet these remain largely underutilised. Traditional data engineering approaches—with their reliance on manual documentation, data mapping, and test scripting—consume disproportionate resources while delivering incremental value at best.
Meanwhile, the financial institutions embracing AI-powered data transformations are creating a widening capability gap:
Data engineers augmented by AI are delivering solutions in days that previously took months
Quality engineering processes are reducing end-to-end delivery costs by 25%
GPU acceleration is delivering performance boosts of up to 640x for critical data workloads
Automated data governance and quality management is dramatically reducing compliance costs
This isn't merely about operational efficiency. It's about fundamental competitive positioning in a market where speed, intelligence and cost-effectiveness will increasingly determine winners and losers.
Beyond the Obvious: The AI Advantage You Haven't Considered
While many executives focus on customer-facing AI applications, the transformative potential in data operations remains largely untapped.
By 2027, the application of generative AI (GenAI) will accelerate the time to value of data and analytics governance and master data management (MDM) programs by 40% Gartner, Magic Quadrant for Augmented Data Quality Solutions, 2025
The emergence of Retrieval-Augmented Generation (RAG) systems now allows engineers and managers to interrogate data and metadata through natural language queries, creating an AI co-pilot that supports data engineers, stewards and analysts in refining transformations and improving lineage.
AI is increasingly augmenting data analyst and steward roles by drafting content, using enterprise metadata, taxonomies and data profiles to provide the insight (e.g. Atlan). Not only does this enhance the curation of the data catalogue, but it also provides a basis for ongoing monitoring.
Some examples include:
Automated Data Classification and Tagging
Data Quality Management (machine learning identifies – and possibly corrects – data inconsistencies, duplicates and errors)
Predictive Data Governance (identify issues based on changes to data)
Enhanced Data Discovery and Cataloguing
Data Privacy Management (identifies sensitive data, applies encryption, and enforces access controls automatically)
Intelligent Metadata Management (generates and updates metadata, improving data discoverability and usability)
Automated Data Lineage Tracking (visualises data lineage, showing data flow and transformations across systems)
Dynamic Policy Enforcement (policies adapt based on regulatory changes)
Fraud Detection and Prevention (identify anomalous data access requests)
Many tools provide one or many of these capabilities already. Hyperscale providers are embedding it into their platforms, including AWS Bedrock Studio and Microsoft Fabric.
Project Aether from NVIDIA exemplifies this hidden opportunity. It uses AI to automatically analyse Spark jobs, identifying optimal candidates for GPU acceleration, then fine-tunes configurations to maximise performance. What it can do in four days would require an entire year manually.
Commonwealth Bank's application of NVIDIA's RAPIDS Accelerator for Apache Spark delivered a staggering 640x performance increase, processing 6.3 billion transactions in just five days instead of the nine years originally estimated. Daily processing of 40 million transactions now completes in 46 minutes with an 80% cost reduction.
These capabilities aren't merely incremental improvements—they fundamentally redefine what's possible.
Most significantly, these aren't future possibilities requiring multi-year investment horizons. One Australian financial services client achieved 4x return on their initial investment within just six months of adopting these tools and practices.
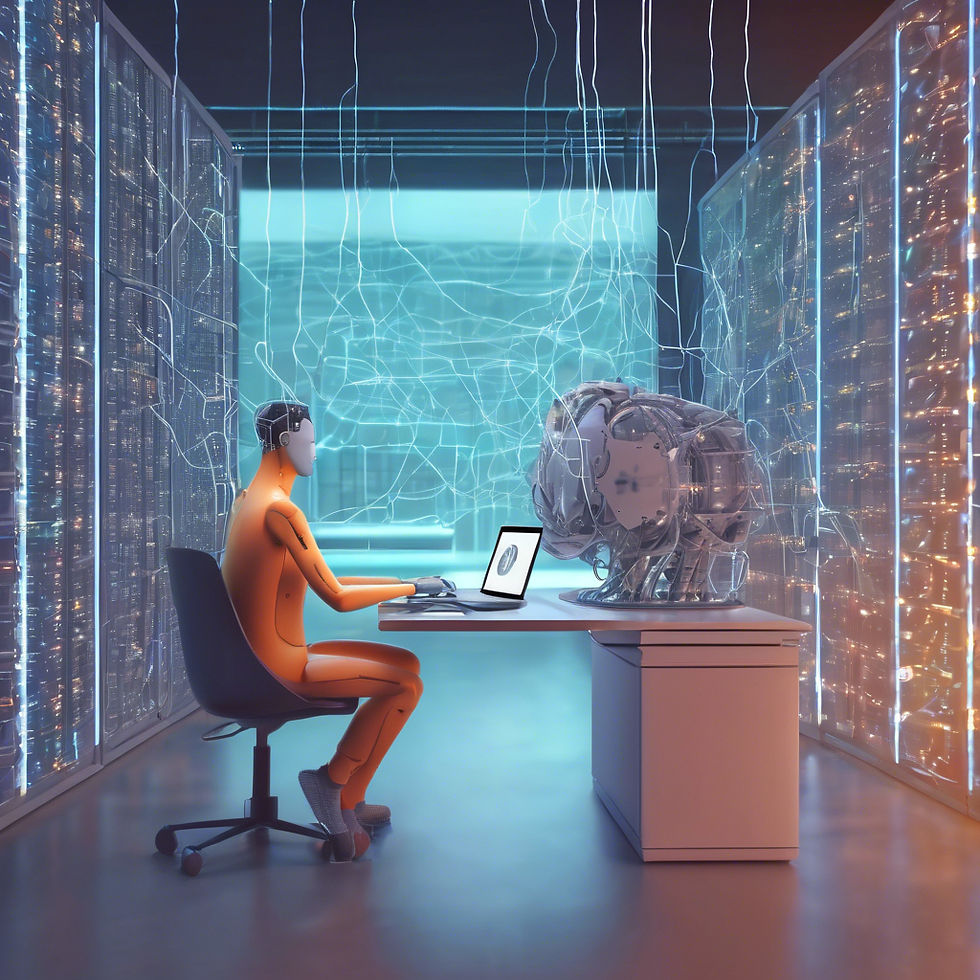
The Burning Platform: Why Tomorrow May Be Too Late
The competitive dynamics in Australian financial services are accelerating at an unprecedented pace. As AI capabilities advance, the gap between leaders and laggards widens exponentially rather than linearly.
This isn't hyperbole—it's market reality:
86% of Chief Data Officers plan to increase investments in data management for 2025, with 44% citing data readiness for generative AI as the primary driver
CBA has entered a five-year agreement with AWS, making it their largest cloud provider, specifically to enhance the computational power supporting their extensive AI model portfolio
Regulatory scrutiny is intensifying, with ASIC's Joe Longo noting that "the volume of AI use is accelerating rapidly, with around 60% of licensees intending to ramp up AI usage"
The implications are clear. The time for cautious experimentation has passed. Financial institutions that fail to aggressively modernise their data operations through AI will face an increasingly insurmountable competitive disadvantage.
"So whatever part of that lifecycle you're thinking about: whether it's ingestion of data onto the platform, whether it's test automation, metadata capture, understanding requirements, or maybe it's legacy logic that you've written, we've been building AI agents for all of that process" Andrew McMullan, CBA Chief Data Officer, Mar 2025
As consumer expectations evolve and regulatory requirements intensify, the question isn't whether your organisation will embrace AI-powered data transformation—it's whether you'll do so while competitive advantage remains attainable.
The Path Forward
The most effective approach isn't a wholesale transformation but a targeted strategy focusing on high-value opportunities:
Adopt Quality Engineering practices to automate the data lifecycle, reducing costs while increasing accuracy
Leverage cloud capabilities to access new tools and increase data processing capacity
Integrate AI into data governance and quality to augment existing initiatives
Establish AI frameworks that properly manage risk while enabling innovation
Implement GPU acceleration if you have high-volume workloads to dramatically reduce cost and throughput times
The financial institutions that thrive in this new landscape won't be those with the most data or the largest technology budgets. They'll be those that most effectively harness AI to extract value from their data assets with unprecedented speed, intelligence and efficiency.
The question isn't whether your organisation can afford to make this transition.
It's whether you can afford not to.
AegisIQ is passionate about making technology a transformation enabler, ensuring it is human-centric and seamlessly integrated into the business. Connect with us today to see how we can help you become future-fit.
Comments